This article is written by Matt Lerner, co-founder at SYSTM, a company that helps startups “find their big growth levers” (and pull them). Previously, he was an investor at 500 Startups and ran B2B growth teams at PayPal. He previously shared an excellent guide to finding language/market fit here on The Review. Today, he’s generously sharing a chapter from his new book “Growth Levers and How to Find Them,” penned for, as he puts it, “impatient founders and their teams.” For more of his insights, check out his 2-minute newsletter.
Every great startup made wrong turns before unlocking hockey-stick growth. How many wrong turns will your startup need?
As a thought experiment, let’s imagine we know for certain that you need to make precisely 1,000 wrong moves, and, after 1,000 failed experiments, attempt 1,001 would be the one that catapults you to decacorn status. If you knew that from Day 0, how would you run your business differently?
You would experiment like crazy. Every team. Ten tests per week — per employee. You’d hire data scientists and maybe even actual scientists. You’d teach everyone to properly set up experiments, find statistical significance, and look out for type I and type II errors. You might even get a tattoo of behavioral scientist Daniel Kahneman.
Of course it’s not 1,000. We can't know the actual number, and it won’t be so binary. But what does that change? Regardless of whether that number is knowable, in the early days of any startup, you need to learn a bunch of things the hard way. Why not do that as fast as humanly possible?
This sounds obvious in theory. Nobody sets out planning to move slowly, waste time on bad ideas, and test nothing. But in reality, we’re all a bit overconfident, so we tend to go all-in on our favorite ideas and only experiment at the margins, rather than experimenting constantly with bold swings.
Back when I was a VC, I saw this pattern over and over again in the go-to-market section of pitches. Instead of focusing on big opportunities, founders were recycling a tired list of basic growth ideas that would never amount to much. Instead of finding big growth levers, they were pulling the small ones harder and hoping for the best.
Great startups don’t waste time on small stuff. Every early-stage company works hard, but great startups prioritize incredibly well.
Here’s what I mean by big growth levers: Early growth at startups comes down to a few insanely successful tactics. If you look back at the early days of any great startup, you’ll see that 90% of their early growth came from 10% of the stuff they tried. Some famous examples:
- Airbnb wrote a script to automatically post each new listing to Craigslist bringing them loads of free qualified renters.
- PayPal wrote a script to message eBay sellers, pretending to be a buyer, to ask if they would accept payments via PayPal. Sellers thought their buyers wanted PayPal, so they quickly added the payment option.
- Canva created thousands of SEO-optimized pages with design templates and tutorials because they realized many basic design tasks start with a Google search like “award certificate template.”
- Facebook and LinkedIn created an onboarding process in which new users automatically invited friends and colleagues to join.
This phenomenon is no coincidence — it’s physics. Big companies can grow by deploying heaps of cash and armies of people, using every channel, hoping one will work, and not really understanding which ones do. But startups with a few employees, a couple million dollars, and 1–2 years of runway don’t have that luxury. They need to find the small actions that bring huge numbers of customers, quickly.
For startups, finding big growth levers is a matter of life or death. Most startups never find them, and they die.
“Find” is the operative word there — this process is fundamentally a search. And in a search, your chance of success depends above all on one factor: the pace and quality of your learning. Reflect back on your team’s work these last several months and the growth experiments you’ve run. More specifically:
- How satisfied are you with your team’s rate of learning? Do they tell you new things about your customers every week?
- How often do they challenge your strategy with new information about the market?
- When an experiment fails internally, how does that conversation go? Does your team talk about what they learned? What they’ll do differently next time? Or are people afraid to tell you about their mistakes?
- Is each misstep making you smarter and moving you closer to success?
Before you can become a “growth machine” you need to become a learning machine. The first overarching goal is to turn your startup into an instrument for discovering your big growth levers. In my new book on growth levers, I recommend three steps:
- Understand your customers using Jobs to be Done
- Map your growth model to help you find points of leverage so you can identify and prioritize the most impactful work
- Rapidly experiment to find what works best.
While I cover the first two steps in the first half of my book — with step-by-step instructions for interviewing your customers and mapping your growth model — the “rapid experimentation” part of the equation is my focus here today, because it all comes down to the pace of learning.
If the stuff you're doing now is not having a big impact, and nobody's learning from it, then it isn't magically going to start working. If you have found a big lever, congrats, focus everybody on it. But if you haven't, then focus on finding one, not just pulling the small ones harder.
To help you accelerate the pace and quality of learning across your whole team, I’ve open-sourced my chapter on running growth sprints below. I’ll cover how they differ from product sprints, how to prioritize your experiment backlog, and the ingredients of a good hypothesis. Importantly, I'll also dig into how to close the loop by extracting learnings, debugging failed attempts, and empowering your team to run faster and bolder tests. Let’s dive in.
THE CASE FOR GROWTH SPRINTS
Product teams run agile sprints with groomed backlogs and acceptance criteria. They’re fast, deliberate, and reflective — nothing happens by accident. But on the go-to-market side of the house? We’re still making annual plans and huge, expensive campaigns. And prioritization is unsystematic. Should we rebrand? Try LinkedIn ads? Online events? TikTok? Influencers?
While product sprints are a well-oiled machine, most marketing is still a slow (and expensive) exercise in trial and error — which is bewildering because eventually every company’s success hinges on customer acquisition.
That’s why if you haven’t already, I strongly encourage you to add on growth sprints as a core company ritual. My preferred approach (which I outline in full below) is a simple 5-step mix of agile and the scientific method, but unlike product sprints, it involves the whole company, not just product and engineering. For example, I’ve seen customer success teams make a big difference here. And back when I was at PayPal, we cracked a $100M churn problem with targeted action from our operations team. Zero product, marketing or engineering — only a spreadsheet, a bit of SQL and a physicist named Ben.
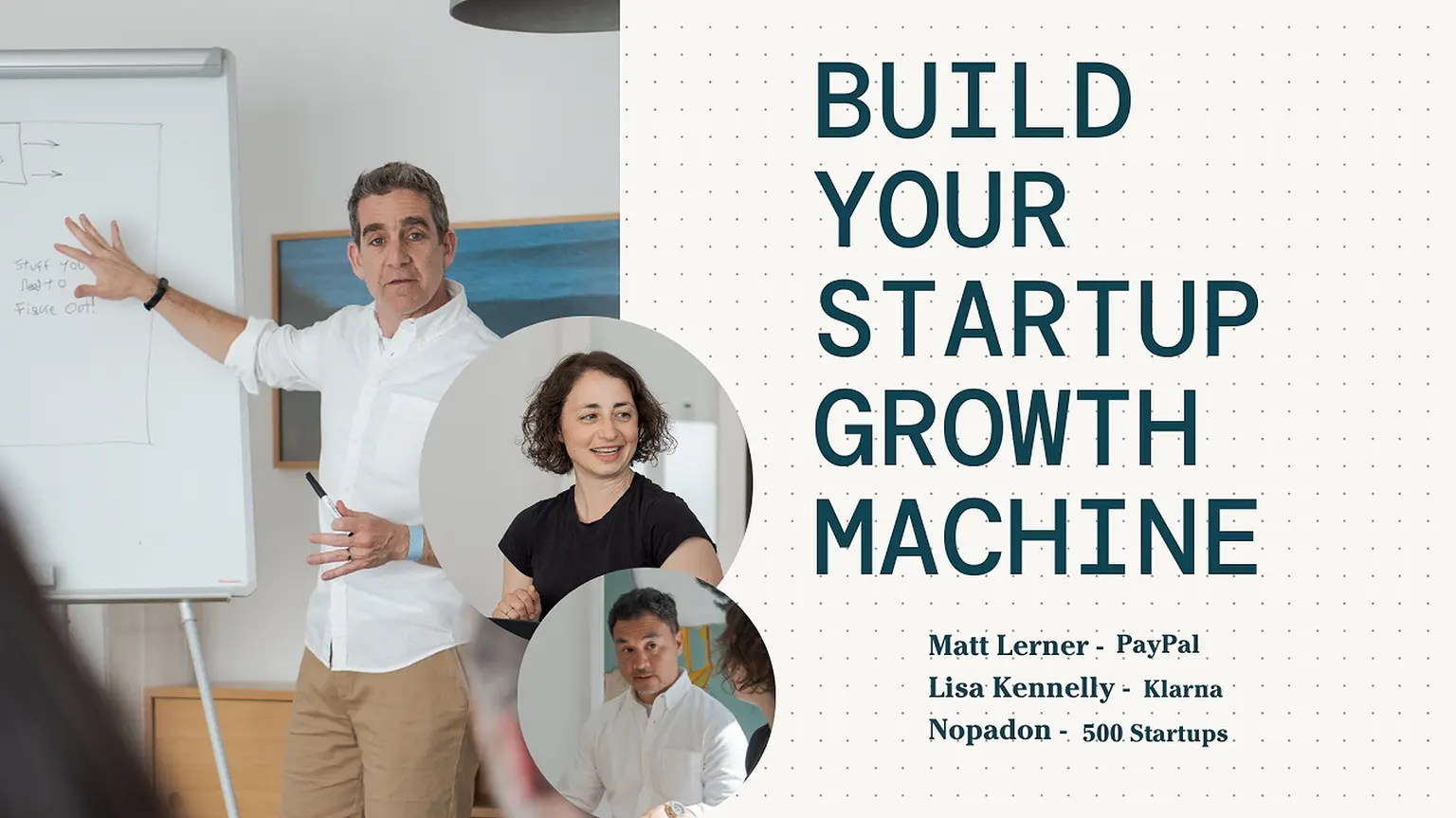
If you like Matt Lerner's advice, consider taking his Build your Startup Growth Machine course on Maven
The growth sprint process has four goals:
- Align the entire company (not just marketing or product) around growth
- Prioritize the most impactful work, with the greatest potential upside (regardless of cost)
- Rapidly test the most promising ideas with experiments to discover what really works, including finding the best messages and channels, and isolating the ideal target customers
- Disseminate what you learned from each experiment to accelerate the pace and quality of learning across the company
Each experiment aims at testing a specific hypothesis. These go beyond simple A/B tests; you can use them to validate new product or feature ideas, possible new customer segments, or even business processes.
This requires rightsizing your expectations, however. Unlike product sprints, most growth experiments fail, so your primary outcome is learning — especially from the failures. If the experiments are well designed, failures will help you eliminate bad options and focus your efforts. With enough persistence, patience, and a bit of luck, you’ll find winners, and your data will give you the confidence to double down on them.
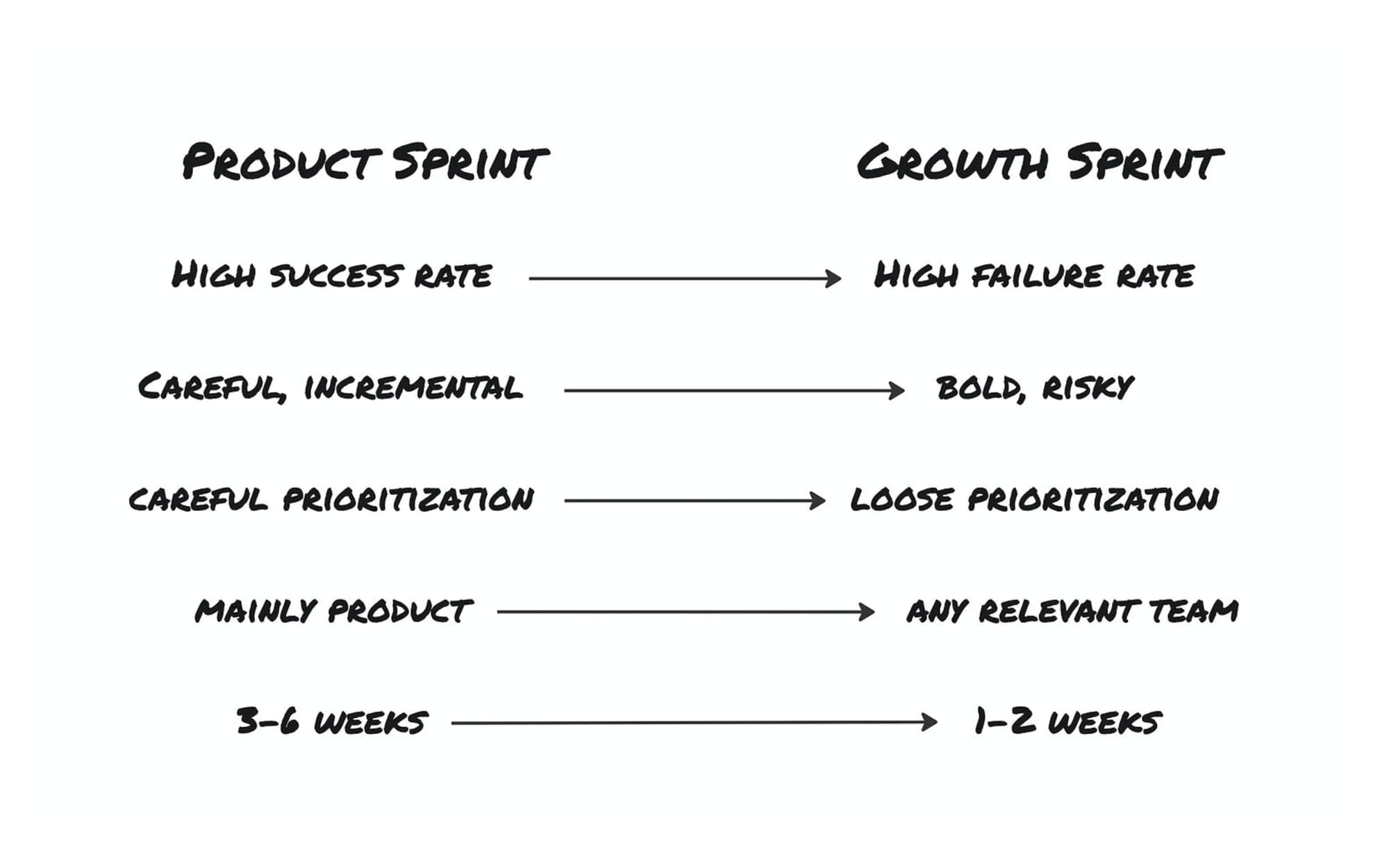
With every growth experiment, you're not just trying to optimize your return on ad spend, lower your CPA, or bag a few new logos ahead of the next fundraise. You're fundamentally learning and making progress toward a simple question: How are we going to grow this business?
To get more granular, I follow these steps when running a growth sprint:
- Inputs: Gather your customer insights and the data in your growth model, plus any past experiments or ideas.
- Ideation and Scoring: Triage your ideas to create a shortlist for consideration in the current sprint cycle.
- Selection: Select the most promising ideas to test.
- Experiment: Design and run your experiments.
- Learn: Learn from the experiments, document, and share your takeaways.
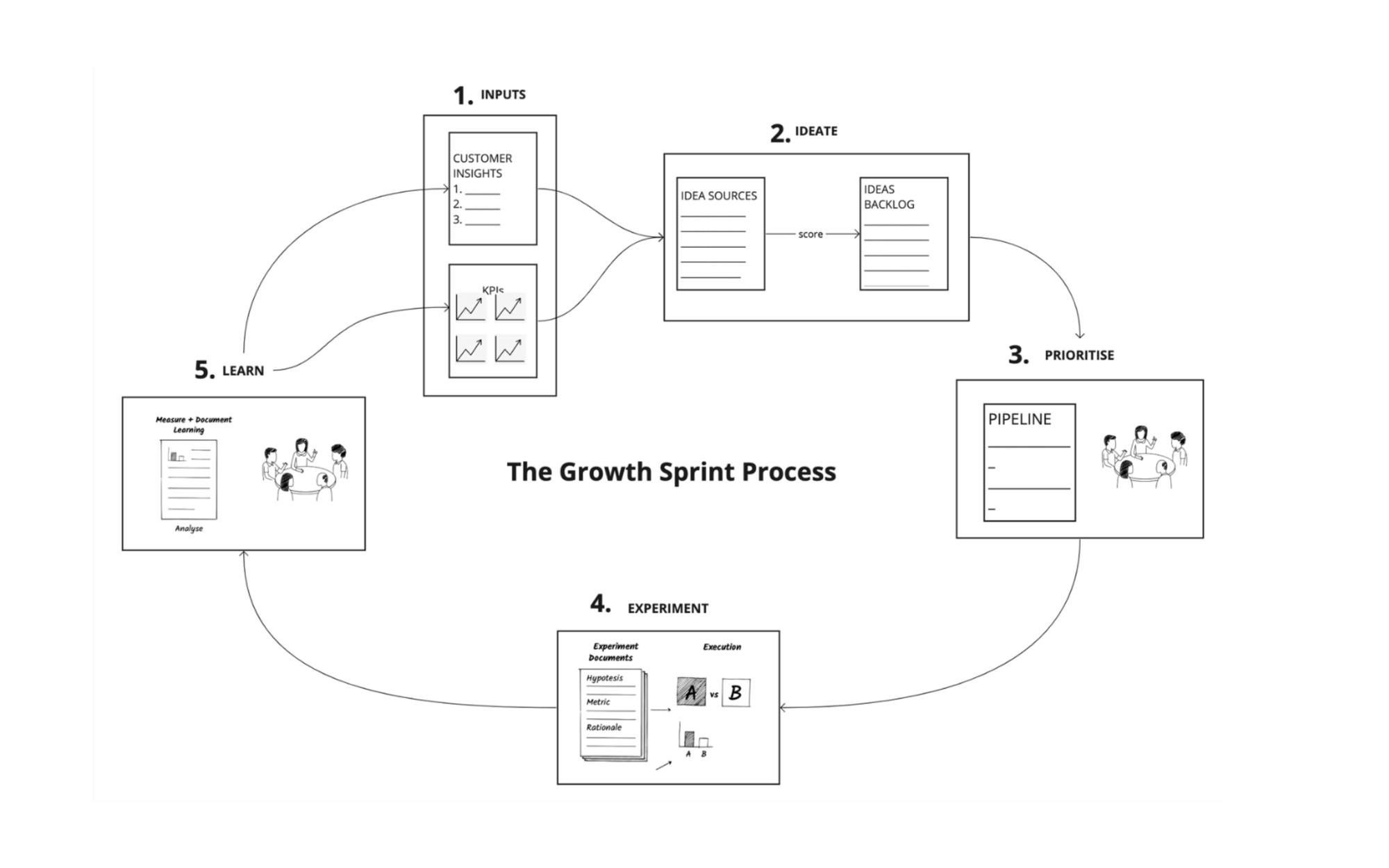
You can adapt the process to the particulars of your business — team, tools, org structure, and culture. Some growth teams need engineers; some don’t. Some companies track growth ideas in Jira, some use Trello, and some use sticky notes. Some teams have growth meetings on Tuesdays, some on Fridays, and some every two weeks. I will cover none of these details.
Instead, I’ll discuss the core principles and goals of the process and the rationale for each step. That way, you can adapt the process to your existing workflows because the best process is the one you’ll actually use.
STEP 1: GATHER YOUR INPUTS
Your big levers are seldom obvious. Generic tactics like paid ads, SEO and referral programs will only get you so far. Growth levers are unique to each startup’s specific, unexpected learnings — so every sound hypothesis for a growth experiment must start with a customer insight.
For our purposes here, I’ll assume you’ve already done the hard work of interviewing your customers and mapping their journey to your product, gathering tons of ideas for experiments in the process. (But if you’re starting from scratch, the TL;DR is that I recommend following the jobs-to-be-done approach to surface customer insights. You can read this quick JTBD primer here on The Review, but Chapters 2-4 of my book will also help you find your way.)
To give you a sense of how customer insights can translate into growth experiments with real results, here are a few examples from companies I’ve worked with:
- Photobook app Popsa figured out that prospects were suspicious of their product category, because photobook apps are notoriously tedious. They changed their tagline from “Fast easy photobooks” to “Photobooks in 5 minutes” and quadrupled installs.
- FinTech app Rebank realized that most of their prospects — all startups — had basic finance questions before they were ready for Rebank. So they published a detailed free guide, “Finance for Founders,” that generated thousands of qualified leads and helped them grow 22X in 18 months.
- FATMAP offers 3D terrain maps for hikers and backcountry skiers. Customer interviews revealed that most trail map searches start on Google. So they published every trail, route and piste in their database in the form of 100,000 keyword-optimized web pages. That brought in an additional 80,000 qualified prospects per month.
- Scheduling app Cronofy discovered five niche use cases among their most loyal customers, like booking HIPAA-compliant medical appointments. They replaced their generic “book a demo” homepage link with those five options, and quintupled their conversion rate.
STEP 2: IDEATION AND SCORING
With your ideas gathered, the next step is a triage: quickly filtering down the backlog of ideas. Your team should have many ideas based on information from customer interviews and your data. You need to sort through them quickly and find a few that can have a huge impact.
Looking at the list of ideas, score each one quickly on three factors:
- Key Driver: Key drivers represent the results of the work people do to move the North Star, but not the work itself. (For example, you could break a measure of weekly active users into three drivers: signups, activations, and retention.) So if a growth experiment you’re considering works, which of your key drivers will it most directly impact? Traffic? Conversion? Retention? Referrals? Activation?
- Impact: If it works, how big would it be, on a scale of 1 to 5? For example, if you’re considering an SEO project, and the search term you’re chasing only gets 10 searches per month, that number limits the potential impact. Or if you’re considering changes to your pricing page, but only 2% of your traffic ever visits the pricing page, that change will only affect 2% of your traffic. (Don’t overthink this, just make a quick order-of-magnitude estimate. It’s very hard to do this accurately, and it’s not worth the extra time and effort.)
- Effort: How much time, effort, money, and engineering would be required? Blend those costs into one number from 1 to 5. Again, estimate quickly, don’t overthink it.
Here’s an example of a growth idea backlog:
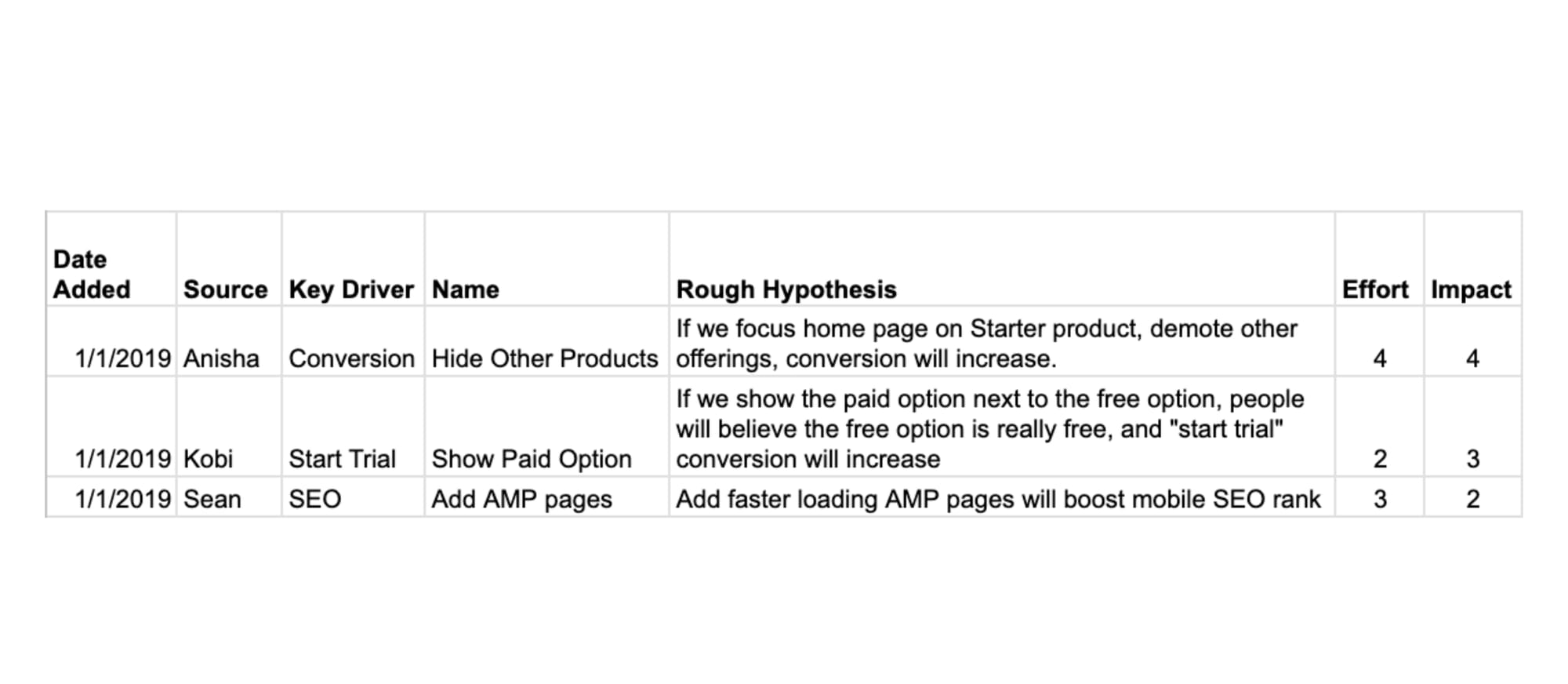
Next, remove ideas that don’t move your key drivers. Among the remaining ideas, start with those that impact your rate-limiting step. I dive into rate-limiting steps more deeply in my book, but essentially, it’s a bottleneck that constrains the overall growth rate of your business. For example, for a meditation app, if 95% of new signups churned after their first time using the app, it would make no sense to invest in finding more traffic, improving conversion on the landing page, or building a referral program because the onboarding experience is their rate-limiting step.
A good way to isolate your rate-limiting step is to ask: “If we double our performance in this area, will we double the business?”
Finally, sort by potential impact, from most to least. You’ll gravitate toward high-impact and low-effort ideas, naturally. But keep the high-effort ideas on the list. We’ll talk about how to test them further down.
STEP 3: SELECTION
The scoring process will shorten your list of ideas, but you’ll still need to choose a few to test each week. If you can test more, great. Run as many good experiments as you can with your resources. Sometimes one test requires engineering, another one is just design and copy and the third is a customer service treatment, so you can run them all in parallel. But if all your ideas are landing page tests, then you’re more constrained. I find that fast-moving teams often set a goal of 3-5 per week.
Here are some prompts you can use to decide which experiments to run:
- Which of these, if it works, could have the biggest impact?
- Will we learn something important about our business or our customers? Will this resolve an internal debate we’ve been having?
- Is there an easier way to get that information, such as studying our existing data, asking customers, or speaking to our salespeople?
- Is the idea actually risky? Could it backfire? Or is it expensive in terms of money, time, engineering work, or some other scarce resource? (If not, maybe just do it rather than run a formal experiment.)
- Do you have the resources and technological capability to run this experiment well?
The more data you have, the easier this decision will be. If you’re finding these decisions difficult and contentious, try to identify the missing information that would make it easier to decide.
STEP 4: EXPERIMENTATION
What’s the difference between an “experiment” and “just do it?” The difference is only about 5 minutes, but it’s an incredibly valuable 5 minutes. That’s the time it takes to write a hypothesis and make a prediction. Documenting your prediction helps clarify your thinking and eliminate hindsight bias. That way, no matter if your experiment succeeds or fails, it will make you a little bit smarter.
Does everything need to be an official experiment? We don’t always have the time, tech, or traffic to run a proper, controlled experiment, and that’s okay. Sometimes, “just do it and see what happens” is a perfectly good approach. But every project, whether you get a clear winner or not, needs to bring learning.
Do the upfront thinking it takes to ensure you learn from your experiments — always start with a clear hypothesis and prediction.
Formulating a hypothesis
Writing a hypothesis only takes a few minutes, and it’s a great way to clarify your thinking. Here’s the format:
- The Risky Assumption: We believe that ______.
- The Experiment: To test this, we will ______.
- The Prediction: We predict that ______ (metric) will move by ______ units in ______ direction.
- The Business Effect: If we are right, we will ______. Think: how will this change the way you run your business? If you can’t think of anything you’d do differently, it’s probably not worth running the experiment.
Start with a hypothesis that contains a single risky assumption that can be tested and measured. For example: “We know that app users have better retention rates than web users, and we believe this is causation, not correlation. Therefore, if we encourage new signups to install our app after they register, rather than using our web version, we predict it will increase our 90-day retention rates from 8% to 12%.”
Avoid bad hypotheses
The most common reason experiments fail is starting with a poor initial assumption. A good assumption contains a belief about your customer that’s rooted in some insights you gained from your data or customer conversations.
A very common mistake is to draft a circular hypothesis like this:
We believe that if we lower our price by 10%, we will get more signups. Therefore, we will offer a 10% discount, and we predict our total sales will increase.
A circular hypothesis basically just says: “I have an idea, I think I’m right, so I’m going to try it, I predict it works.” Testing them doesn’t help us learn.
If you ran that 10% discount experiment and it failed, what would you do next? Lower the price more? Revert to the original price? Raise the price? And if the experiment succeeded, what would you do next? Lower the price further? Keep the discount and move on to another unrelated experiment? It’s hard to come up with a next step because this experiment hasn’t tested any assumptions about your customer.
A better hypothesis would focus on an assumption about our customers’ beliefs that we wish to clarify or validate. For example, in the case of our 10% discount, what customer beliefs would need to be true for a 10% discount to be a good idea?
Perhaps we believe most of our prospects like our product but aren’t buying because it’s slightly too expensive. Therefore, if we lower the price by 10%, it would be within their acceptable price range. If that were true, then a 10% discount would be a great idea.
Alternatively, maybe we believe most prospects like our product but lack urgency around the purchase. Therefore, if we offer a small discount with a deadline, we can use that deadline as a forcing function to move prospects across the line. The truth is both of those hypotheses sound kind of basic because this 10% discount idea was a generic tactic, not rooted in any real customer insights.
Therefore, as you draft your hypothesis, start with an assumption about your customer rooted in your customer interviews, like these examples:
- We believe prospects are not buying our product because, based on our home page headline, they do not understand how our product will help them. Therefore, we will test versions of our headline that more clearly describe, using actual customer language, the top outcomes that a prospect can expect from using our software.
- We believe prospects are not clicking “book a demo” because they are still in the information gathering and comparison stage of the purchase journey, not yet ready to speak with a salesperson, as “book a demo” implies. Therefore, in addition to “book a demo,” we will offer visitors the option to download a buyer’s guide to help them understand our product category so they can feel like they’re making a more informed decision.
- We believe prospects are not downloading our "buyer’s guide” because they think it’s biased in our favor. Therefore, we’ll commission a respected independent industry expert to author our buyer’s guide and create a visual design that does not reflect our branding.
- We believe prospects are not signing up for our product because they are worried it won’t work. Therefore, we will surround the “sign up” button with customer testimonials that call out people’s specific results, and we will highlight our money-back guarantee.
You’ll notice these are all negative hypotheses (e.g., “We believe prospects are not doing X because…”). A negative phrasing reduces the risk of writing a circular hypothesis. Statistical researchers always try to disprove a null hypothesis (e.g., we believe income and education are not correlated). Doing so reduces the risk of confirmation bias.
Most growth experiments aim to convince people who aren’t doing a certain thing to start doing it. Ideally, those experiments rest on a theory about why they’re not doing it in the first place.
Next, describe your experiment at a high level: “We will randomly split new signups and redirect half of them to the app store, and allow half of them to stay with our current mobile web experience.”
Then, choose a success metric that’s deep enough in the funnel to be meaningful (e.g., not “clicks”) but shallow enough to get your data quickly. For the app retention experiment, maybe your primary metric is one-month retention rates. But also keep an eye on the number of logins, transactions, or some other behavioral metric, and track long-term retention metrics as the cohort matures.
Place your bets
Now, make a specific numerical prediction. For example:
“We predict that month 2 retention increases from 38% to 50%.”
You need a specific numerical prediction because your estimate of the impact will be used to calculate your sample size. Pick a big target. If the experiment won’t have a big impact, it’s not worth your time.
Finally, consider the business effect. What action(s) will you take if your test is successful? For example, maybe you’ll create and launch an Android version of the app and push new signups to install the app. Also, what if the test fails? Maybe you’ll remove the interstitial that sends people to install the app.
Write down your hypothesis and share it with as many team members as you can. Encourage them all to make predictions or even “bets,” giving a prize to the winner. It’s a fun competition that helps everyone learn about the business, your customers, and the experimentation process in general. It also reduces the chance of people saying “I knew that all along” when the results come in. Instead, they’ll have to reconsider their past assumptions.
Run small tests of big ideas
You’ll quickly test your “high impact, low effort” ideas, but what about those high impact and high effort ones? Often, the best ideas are hard or expensive, but that’s no reason to rule them out.
If you don't test big things, you won't find big levers.
When working with startups at SYSTM, we encourage teams to start with the biggest-impact ideas — regardless of cost or effort — and dream up ways to test them with small, quick experiments. That’s the secret: small tests of big ideas, or minimum viable tests (MVTs). (My friend Gagan Biyani, founder and CEO of Maven, wrote an excellent guide to MVTs here on The Review that goes quite deep, but read on for my take below.)
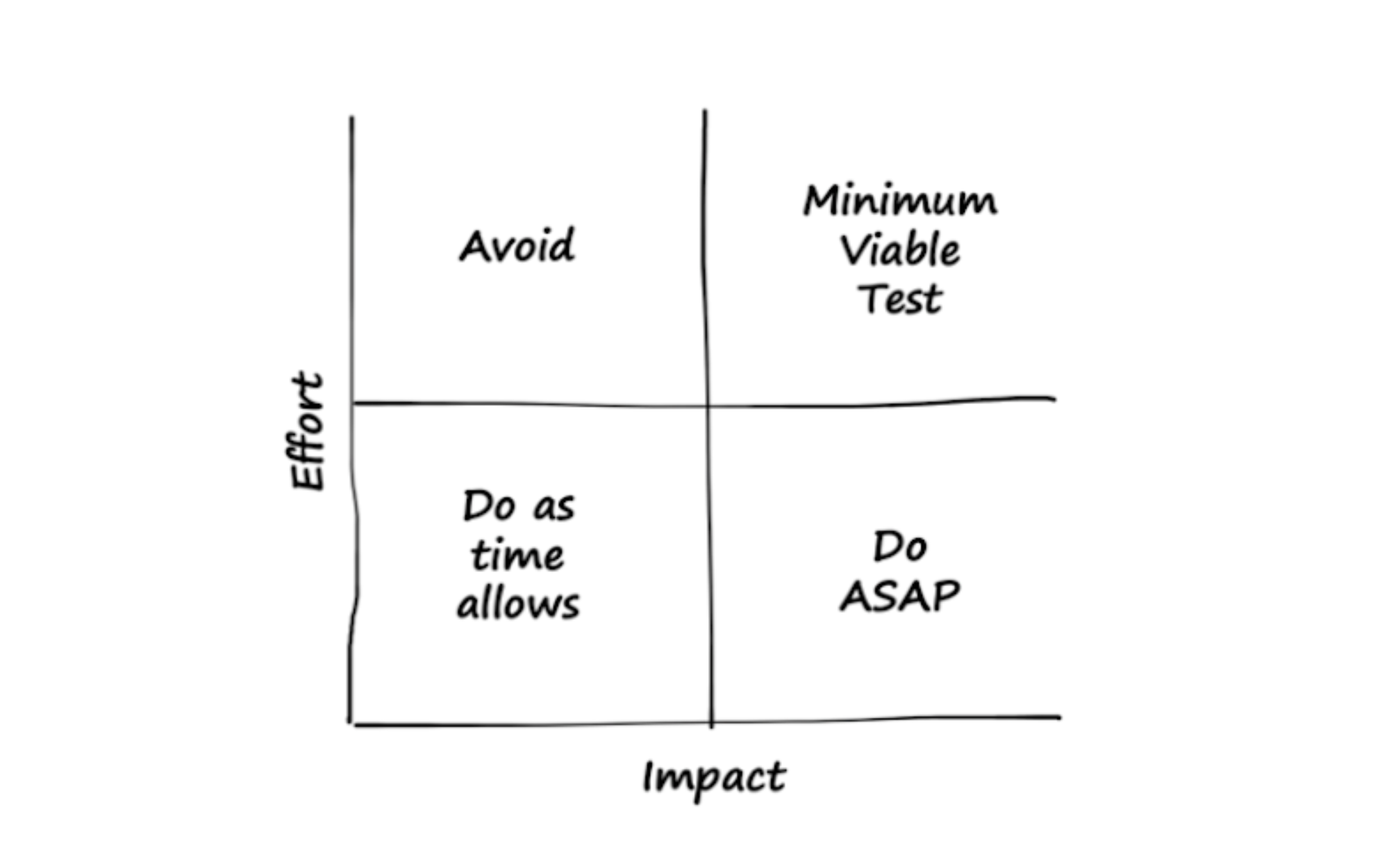
Consider these two examples:
- Airbnb’s founders suspected better photography might improve rental rates. But sending professional photographers to every property would have been complex and expensive. Fortunately, Airbnb was founded by design students, so they grabbed their cameras and spent a weekend photographing only the listed apartments that were near their base in New York City. They found their professionally photographed listings rented faster and for higher prices. That result justified a larger rollout with professional photographers across their properties.
- An ecommerce app was debating adding PayPal to their checkout flow, but the integration would’ve required scarce engineering resources, and PayPal was more expensive than Stripe. To quickly test the idea, they added a PayPal button to their mobile web checkout flow without actually enabling PayPal. (If people clicked it, they were prompted to enter their credit card details). They found that about a quarter of their shoppers clicked the PayPal button, and many of them abandoned the checkout when they realized they could not actually use it. This gave the team the data they needed to justify adding a PayPal option.
It’s fine to score growth ideas based on effort and impact. But don't disregard the “high effort” ideas. Instead, find a quick cheap easy way to test them.
If you have a big idea that’s hard to execute, instead of testing the whole idea, you’ll start by quickly testing only your riskiest assumption. Start with a pre-mortem listing all of your risky assumptions.
Imagine that the project has failed, and list all the possible reasons it could have failed. Most risky assumptions fall into these three categories:
- Lack of customer interest: Mistaken assumptions about what our customers want or how they would behave.
- Poor execution: Some things are harder to execute than we assumed, either experimentally or at scale.
- Small market: Some ideas can be executed, and customers love them, but the opportunity is smaller than we realized.
If you’re stuck here, your investors can probably help you spot your risky assumptions — they’re rather good at anticipating what might go wrong. After you’ve listed all your risky assumptions, try to narrow down the list to the single riskiest one. Then, write your hypothesis and design an experiment to isolate and test that particular assumption rather than the whole idea.
Thinking honestly and clearly about risks is a rare and useful skill.
Here are some examples of clever MVTs:
- Landing page test: Before you build the product, create a landing page, buy some qualified traffic to it, and see if anyone clicks “buy” or “sign up.” (If they do, apologize that your product isn’t ready and add them to your waiting list.)
- False door test: Instead of adding a product feature, add a button for the feature and see if anybody clicks it. If they do, apologize and let them know the feature is not yet available. Maybe ask them what the feature would enable them to do?
- SEO: An effective inbound / content / SEO strategy can take a very long time. But you can validate the idea quickly with a keyword research tool. Take your search terms and check how much traffic they get each month, how competitive the term is, and which pages currently rank. Are there many searches? If you create good content, could you rank in the top three? Next, build a landing page and pay for some traffic to see if it brings you good-quality prospects.
- Concierge: Before you build a complex product or feature, try delivering the service manually. For example, I worked with an early-stage startup developing a training app. They hoped adding notifications would boost usage, but they had a long product roadmap and only one engineer. The co-founders downloaded a list of all users and their phone numbers, and randomly sent WhatsApp reminders to half their users, leaving the other half alone. The half who received the messages activated 450% more often than the control group, and building notifications became an easy decision.
STEP 5: LEARNING FROM EVERY EXPERIMENT
If the experiment works, great! Take the time to understand why it worked, and think about what you’ll do next. But most of your experiments will fail — or at least surprise you. (If they don’t, you’re not being bold enough.)
Take the time to look through the data, note any surprises, and discuss the results with your team. Each team member understands different parts of the puzzle. Some are experts on tech. Others are closer to customers, the data, or “best practices” in a field like product or marketing. Bring all their knowledge together.
Here are some prompts for your discussion:
- Did anything unexpected happen?
- Any theories as to why that happened?
- What did we learn about our customers?
- What did we learn about our proposition?
- What did we learn about our own thinking / assumptions?
- What did we learn about our ability to execute?
- What did we learn about our tools and process?
- Were any new questions or hypotheses uncovered?
- Is there anything we should start or stop doing?
And here are some prompts to consider the next steps:
- Is there any value here? Should we expand, repeat, or scale this?
- Is there anything we should now stop doing or cross off the list?
- Are any follow-up experiments needed?
- What implementation details should we change?
- Do we need to change any of our KPI definitions?
- Who else needs to know about this result?
- Are there any operational takeaways about how to run experiments better in the future, e.g., tracking or experiment setup?
How to debug a failed experiment
If your post-mortem discussions are full of debate and speculation, your experiments aren’t making you smarter. A scientist or engineer would analyze an unexpected result piece by piece to isolate the problem. You can do the same: Step through the whole experiment, isolating each possible point of failure.
For example, maybe you ran a paid social ad that pointed to a landing page with a sign-up offer and got very few signups. Here are some possible failure points:
- Targeting: Did the test target legitimate prospects?
- Attention: Did the test catch their attention? Or did they scroll past it to get to the next zany pet video?
- Readability: If they saw it, was it clear and readable enough? Did they literally parse the words?
- Comprehension: If they read the words, did they interpret them correctly? Do they understand what you’re saying?
- Trust: Even if they understood your promise to help them, did they believe you?
- Resonance: If they understood the words, did they care? Were they able to connect it to something important in their life, like a struggle, goal, or question they’d been pondering?
- Cost / benefit trade-off: If they were excited about your proposition, was it worth the price? Or was the next step a bridge too far either in terms of financial cost, user experience pain, or even unanswered doubts and fears about your product or category?
You can isolate any one of these causes. For example, you could rule out the Targeting variable by paying a premium for AdWords that targets super high-intent traffic. And you could test Readability by showing your ad to people briefly (e.g., for 3 seconds) and asking, “What did that say?”
A campaign could fail for many reasons. Better to isolate and test each one.
When experiments fail, the root cause is often a muddy hypothesis. So, for the next experiment, get very clear on your written hypothesis and success metric. Isolate one variable (e.g., message or targeting or price) and track the right metric. Or try testing bolder things that challenge your most important beliefs and assumptions about your customers. How can you get your team to be more bold? That brings us to the most important issue of all, mindset.
BOLDER & FASTER: FOSTERING THE DISCOVERY MINDSET
You can run experiment after experiment, but you’ll struggle to get a big lift unless you have the intellectual honesty to examine your beliefs critically and allow your riskiest assumptions to be tested. The process doesn’t work without a discovery mindset.
My team and I have worked with over 200 startups. Some learn quickly, others are painfully slow. The difference — every time — stems from the leaders’ mindsets. More specifically, it’s the ability to shift from feeling confident that you have the right answer to feeling confident you can figure it out.
People don’t like being wrong, it’s scary and uncomfortable. When something doesn’t work, that’s the moment of truth. You need to show up and encourage and praise the learning. Time and again, we see that the most successful teams are the ones who have the confidence to view new information as an opportunity rather than a threat. The discovery process ends with growth, but it starts with learning.
If you liked this article, check out Lerner’s book, Growth Levers and How to Find Them, available worldwide on Amazon, Kindle and Audible.